B Eng Student at IGeA UCLM
MINERAL EXPLORATION THROUGH THE USE OF ARTIFICIAL INTELLIGENCE METHODS.
B Eng student and fellowship holder at University of Castilla-La Mancha (UCLM) - 2020: Daniel Carrasco Pardo 1
Supervisor: Pablo León Higueras Higueras 1,2
Co-Supervisor: Julio Alberto López Gómez1.
1: ETSIA-UCLM, 2: IGEA-UCLM
Predictive maps have become a very powerful tool for mineral exploration. These maps are usually created using information such as known deposits and signs of mineralizations locations, soil geochemistry campaigns, geology of the area of interest, etc.
When generated, these maps highlight those areas with the highest potential, making it easier to decide which ones must be studied with more detail and which must be dismissed, lowering therefore the operation cost.
Thanks to the development in the field of artificial intelligence, it is now possible to create such predictive maps using machine learning models, which are software models capable of learning on their own from the data. These artificial intelligence models find patterns in the information they are provided with.
Using these techniques, it is possible to obtain more accurate predictive maps to work with. These maps will be able to consider the relationships between data of very different kinds (images, numbers, words, etc).
Our team is currently working on two projects in the southern sector of the Central Iberian Zone; in this area we can find very well-known mercury (Hg) mineralizations, with no mining interest at the present time, and also antimony (Sb) mineralizations, which is now considered a critical raw material by the European Union.
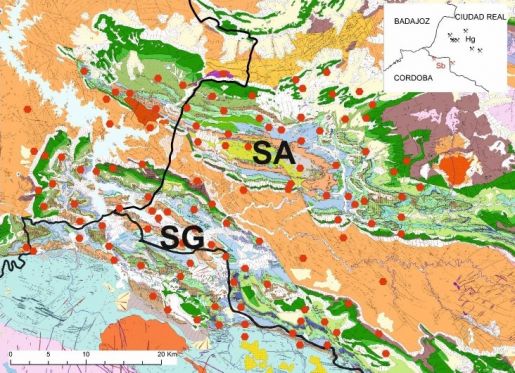
The Almadén syncline hosts the Hg mineralizations while the Guadalmez syncline, which is not far, hosts the Sb ones. Being aware of the geochemical affinity between both elements, and the geographic proximity, we are using the Hg data from the Almadén syncline to train artificial intelligence models capable of creating predictive maps. Once we achieve these models that work well on Mercury mineralizations, the models employed will be applied to create a model for Sb mineralizations, in the context of the southern Central Iberian Zone.
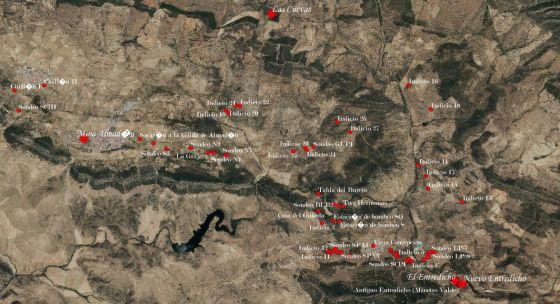
The information we are training the model with consists of:
- Coordinates of the known Mercury Mines, signs of mineralization and drill-holes (77 spots).
- Geological map of the area.
- Soil geochemistry campaign with more than 18.500 samples.
- Further information of each known mineralisation, in the shape of a matrix with 24 columns and 25.000 rows.
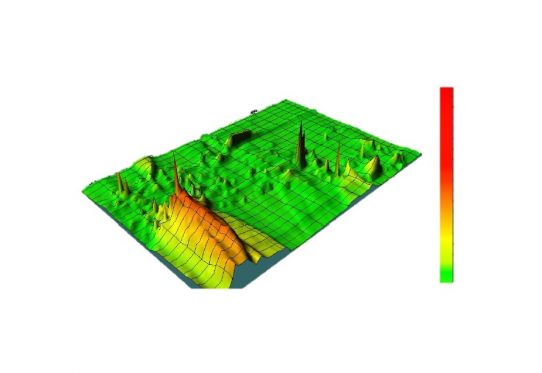
These artificial intelligence models can be the foundation for the creation of this type of maps in other regions of central and southern Europe, in which the exploitation of metals has been very important in recent times.
If the possibility of changing the target element and still obtaining good results becomes a reality, this study could prove that the same model can be adapted to prospect different elements, which will make artificial intelligence an extremely helpful tool for mineral exploration.
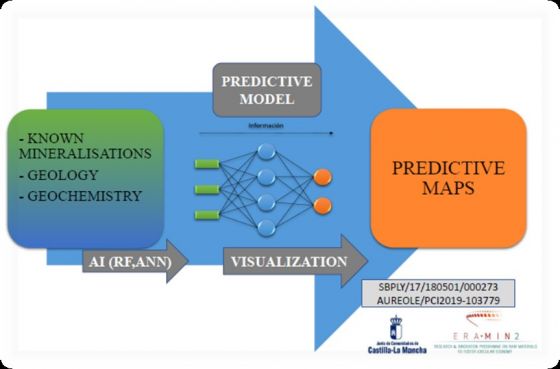
References:
EC, European Commission (2017). Communication from the commission to the European parliament, the council, the European economic and social committee and the committee of the regions on the 2017 list of Critical Raw Materials for the EU. EUR-Lex - 52017DC0490. Available at https://eur-lex.europa.eu/legal-content/EN/TXT/PDF/?uri=CELEX:52017DC04….
Gumiel, P. (1983). Metalogenia de los yacimientos de antimonio de la Península Ibérica. Tecniterrae, 54, 120 pp.
Hernández, A., Jébrak, M., Higueras, P., Oyarzun, R., Morata, D. y Munhá, J. (1999). The Almadén mercury mining district, Spain. Mineralium Deposita, 34: 539-548.
Rodríguez-Galiano, V., Sanchez-Castillo, M., Chica-Olmo, M. y Chica-Rivas, M. (2015). Machine learning predictive models for mineral prospectivity: An evaluation of neural networks, random forest, regression trees and support vector machines. Ore Geology Reviews. 71: 804–818.
Sun, T., Chen, F., Zhong, L.X., Liu, W. y Wang, Y. (2019). GIS-based mineral prospectivity mapping using machine learning methods: A case study from Tongling ore district, eastern China. Ore Geology Reviews. 109:26–49.