PhD Thesis Defense - Alex Vella - 14 nov. 22 - 2 pm, Amphithéâtre ISTO, Orléans
PhD Thesis defense
Highlighting mineralized geological environments through a new
Data-driven predictive mapping approach
by
Alex VELLA
14 nov. 22 - 2 pm, Amphithéâtre ISTO, Orléans
Mineral Prospectivity Mapping (MPM) aims at outlining areas with the highest likelihood for hosting mineralization. In that frame, Data-driven MPM approaches aims at identifying such favorable areas based on features associated to known or studied mineral deposits. Meanwhile, machine learning clustering methods are able to perform a generalization of complex geological environments and features. By combining those methods, with the use of Geographic Information System (GIS) based on spatial and statistical processes, it should be possible to highlight the relationships between known mineralization and the geological surrounding features. However, the input cartographic datasets of Data-driven MPM approach requires pre-processing and homogenization, as they can be of various spatial resolutions and precision, while the use of geological maps can also lead to a misestimation of the relative proportion of geological features, depending on their structural configurations, as maps display 3D structures on a 2D surface. As such, a Data-driven approach, based on the application of a pre-processing method combined with machine learning predictive modeling and clustering methods is developed in this thesis to automate the identification of the relationships between mineralization occurrences and geological features s.l. This method, referred to as the DBA / RF / HCPC method, allows (i) identifying spatial associations of features from the input dataset with the Disc-Based Association (DBA), (ii) quantifying the likelihood of each local association to contain the targeted commodity based on a Random Forest (RF) predictive model and (iii) discretizing the area with the highest likelihood depending on the associations it contains using the Hierarchical Clustering on Principal Component (HCPC). The focus on a Data-driven method allows avoiding as far as possible the influence of known and established metallogenic models to test the effectiveness of “fully” automated processes. Indeed, this approach allows the identification of one or several specific geological environments associated to mineralization within a study area which can easily be interpreted as one or several distinct metallotects for the deposits. In well studied areas, the spatial and statistical relationships found by the model can be compared to the known metallotects and metallogenic models and be used to (i) precise those metallotects (i.e. quantifying weights for its constituting features) and (ii) eventually highlight new metallotects even. Such approach also promises to be very effective for exploration within less studied geological domains giving keys on one to several geological environments to explore, in addition to the delineation of favorable areas. This newly developed methodology is applied as benchmark to the Ibero-Armorican Arc (IAA, Western European Variscan Range) and the relationships between the Variscan structures s.l. and antimony mineralization. We infer this new Data-driven approach applied to natural cases will improve prospectivity mapping and automatic recognition of new metallotects while giving new insights on the genetic processes forming deposits.
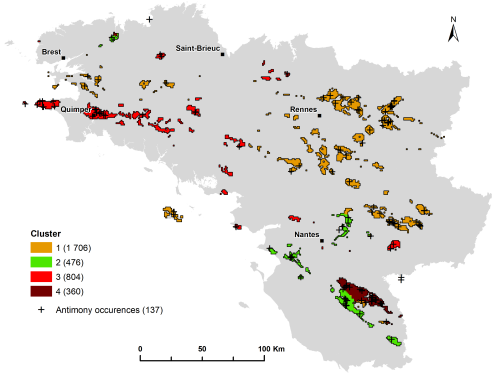